The recent advances in AI (Artificial Intelligence) are transforming human lives. AI-based services are pervasive in the world around us. We use them in our daily lives, often unknowingly.
We ask our voice assistant to check our schedules, tell us the weather, play music, set reminders and even book cabs and shop from our lists. Online shopping sites recommend products that have the highest likelihood of purchase based on our shopping behaviors. Self-driving cars are getting real. This immense proliferation of AI in our daily life use cases is further accelerated by ML(Machine Learning) to efficiently and effectively perform analysis, predictions and decision-making by learning from large datasets.
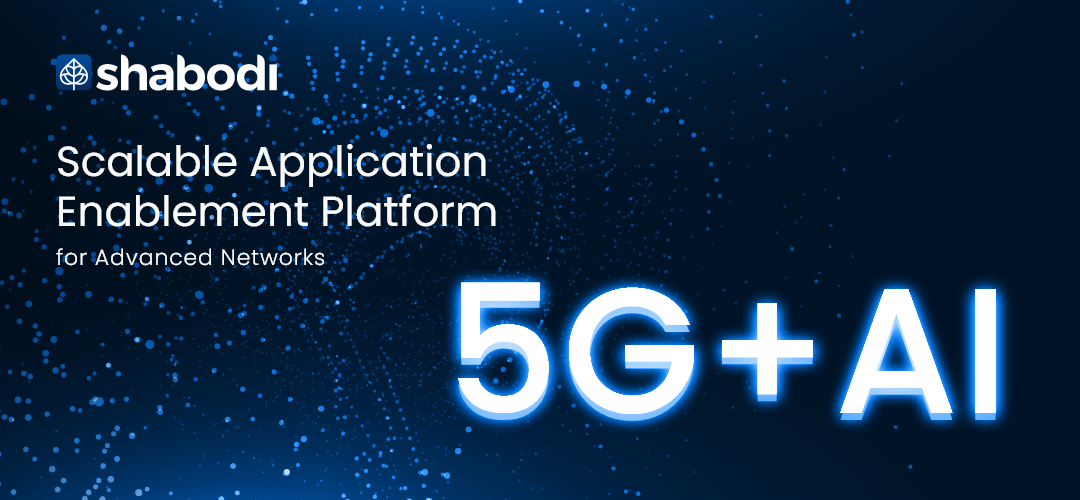
The 5G network is quickly incorporating AI and ML interventions to deliver hyper-intelligent services to end users. In the previous generations of networks, improving user experiences required monumental effort. Much of this work was in gathering data from individual network nodes and operators analyzing it manually to manipulate QoS parameters. This was a reactive approach after instances of network issues had already occurred. Data analysis advantages in such scenarios were reasonably restricted. What users and enterprises needed was the network operator’s ability to provide seamless QoS guarantee without intermittent drops or down times.
NWDAF is a new standard for network analytics in the 5G core defined by 3GPP for service providers to enhance experiences for end-users.
It collects data from 5G core network functions (NFs), user equipments(UE), and operation, administration and management (OAM) systems (Figure 1). Then, the NWDAF performs statistical or predictive analytics with AI and ML algorithms based on collected data.
To address these connectivity and user experience issues, the 5G has a new network function – NWDAF (Network Data Analytics Function). It automatically and in real-time identifies and solves network problems. Further, NWDAF optimizes performance using AI and ML with massive data produced and collected from the network. NWDAF can perform predictive analysis and make proactive decisions without human intervention.
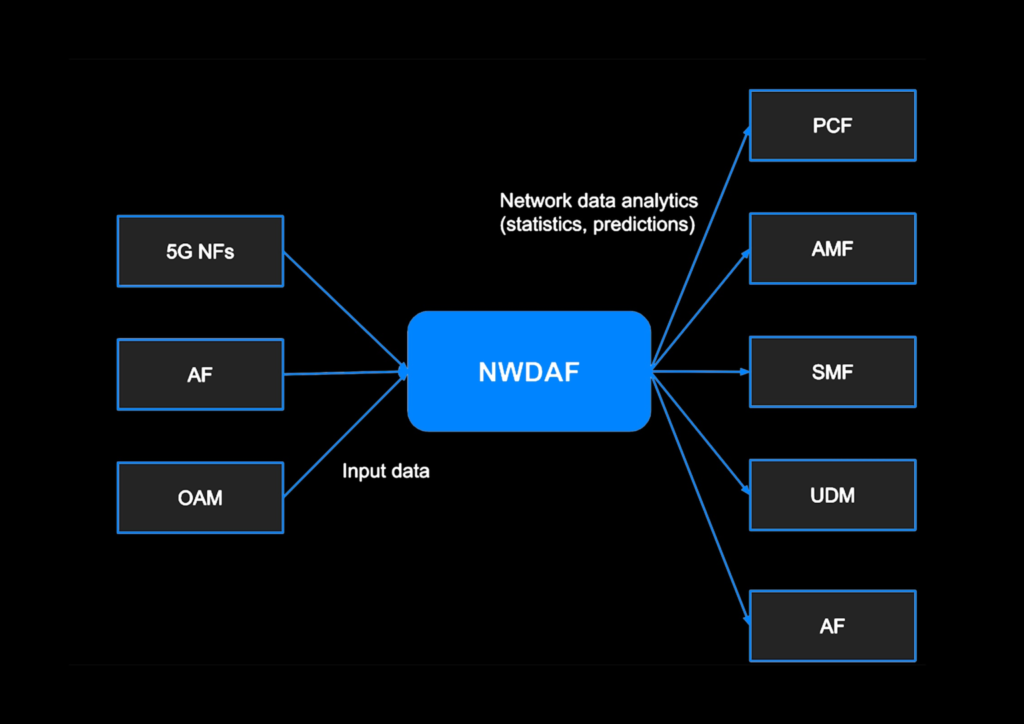
The result derived from AI and ML is used to provide intelligent services to consumer 5G network functions so that the 5G can apply policy and decision making for these NFs in the control plane. The table below describes what services each consumer NF can achieve with network data analytics from NWDAF.
Consumer NF | Intelligent Services |
---|---|
PCF (Policy Control Function) | Calculate and update the authorized QoS for a service dataflow. Determine suitable background data transfer policies that fulfils network performance requirements. Make different policies for abnormal behaviour, e.g., suspicion of DDoS attack to request the SMF to terminate the PDU session |
AMF (Access and Mobility Management Function) | Based on the SMF load, select a suitable SMF during the PDU Session Establishment Optimize the UE paging strategy or learn expected UE behaviour parameters for driving appropriate MICO mode configuration |
SMF (Session Management Function) | Based on the UPF load, select a suitable UPF during the PDU session establishment. Based on UE moving trajectory, select a suitable UPF during the PDU session establishment. Based on the UE communication analytics, select a suitable UPF during the PDU session establishment |
UDM (Unified Data Management) | Store the UE mobility analytics and provision it to the AMF to monitor the UE’s mobility behaviour. Store the UE communication analytics and provision it to the SMF to monitor the UE’s communication behaviour. |
AF Interfacing through NEF | The NEF could provide the user data congestion analytics to the AF to optimize the application information |
V2X Application (AF) | Use the analytics for the purpose of adjustment of the application (adjust inter-vehicle gap, change video codec parameters, etc.) |
NWDAF use cases for MNOs
AI and ML powered NWDAF promises several impactful use cases for mobile network operators. These include mission-critical implementations like detecting congestion or performance anomalies, abnormal behaviour and anomaly detection of UE and service experience prediction for an application.\n
The 3GPP release 16 published in 2020 identified several NWDAF use cases:\n
- Load level computation and prediction for a network slice instance
- Service experience computation and prediction for an application or UE group
- Load analytics information and prediction for a specific NF
- Application service experience computation and prediction
- Network performance computation and prediction
- UE expected behaviour prediction
- UE mobility analytics and prediction
- UE communication pattern prediction
- UE abnormal behavior or anomaly detection
- Current and predicted congestion for a specific location, device or group of devices
- Quality of service (QoS) sustainability – reporting and predicting QoS change
Ultimately, NWDAF enables network operators to deliver on the real power and potential of 5G: uninterrupted, seamless, and radically high connectivity speeds.
Summing up
5G adopting AI and ML innovates monitoring, analyzing and predicting network performance, device behaviours and service experience significantly when compared with previous generation networks.
NWDAF will be one of the most vital enablers of 5G’s impact on intelligence and insights for data-driven network operations and service assurance.